Publications
Statistical physics of complex information dynamics
Arsham Ghavasieh, Carlo Nicolini, Manlio De Domenico
Physical Review E arxiv:2010.04014, ()
Abstract
The constituents of a complex system exchange information to function properly. Their signalling dynamics often leads to the appearance of emergent phenomena, such as phase transitions and collective behaviors. While information exchange has been widely modeled by means of distinct spreading processes–such as continuous-time diffusion, random walks, synchronization and consensus–on top of complex networks, a unified and physically-grounded framework to study information dynamics and gain insights about the macroscopic effects of microscopic interactions, is still eluding us. In this article, we present this framework in terms of a statistical field theory of information dynamics, unifying a range of dynamical processes governing the evolution of information on top of static or time varying structures. We show that information operators form a meaningful statistical ensemble and their superposition defines a density matrix that can be used for the analysis of complex dynamics. As a direct application, we show that the von Neumann entropy of the ensemble can be a measure of the functional diversity of complex systems, defined in terms of the functional differentiation of higher-order interactions among their components. Our results suggest that modularity and hierarchy, two key features of empirical complex systems–from the human brain to social and urban networks–play a key role to guarantee functional diversity and, consequently, are favored.
Scale-resolved analysis of brain functional connectivity networks with spectral entropy
Nicolini C., Forcellini G., Minati L., Bifone A.
Neuroimage (2020)
Abstract
Functional connectivity is derived from inter-regional correlations in spontaneous fluctuations of brain activity, and can be represented in terms of complete graphs with continuous (real-valued) edges. The structure of functional connectivity networks is strongly affected by signal processing procedures to remove the effects of motion, physiological noise and other sources of experimental error. However, in the absence of an established ground truth, it is difficult to determine the optimal procedure, and no consensus has been reached on the most effective approach to remove nuisance signals without unduly affecting the network intrinsic structural features. Here, we use a novel information-theoretic approach, based on von Neumann entropy, which provides a measure of information encoded in the networks at different scales. We also define a measure of distance between networks, based on information divergence, and optimal null models appropriate for the description of functional connectivity networks, to test for the presence of nontrivial structural patterns that are not the result of simple local constraints. This formalism enables a scale-resolved analysis of the distance between a functional connectivity network and its maximally random counterpart, thus providing a means to assess the effects of noise and image processing on network structure. We apply this novel approach to address a few open questions in the analysis of brain functional connectivity networks. Specifically, we demonstrate a strongly beneficial effect of network sparsification by removal of the weakest links, and the existence of an optimal threshold that maximizes the ability to extract information on large-scale network structures. Additionally, we investigate the effects of different degrees of motion at different scales, and compare the most popular processing pipelines designed to mitigate its deleterious effect on functional connectivity networks. We show that network sparsification, in combination with motion correction algorithms, dramatically improves detection of large scale network structure.

Paper submitted and/or under revision
Thermodynamics of network model fitting with spectral entropies
Nicolini C., Vlasov V., Bifone A.
Physical Review E arXiv:1801:06009v1, ()
Abstract
An information theoretic approach inspired by quantum statistical mechanics was recently proposed as a means to optimize network models and to assess their likelihood against synthetic and real-world networks. Importantly, this method does not rely on specific topological features or network descriptors, but leverages entropy-based measures of network distance. Entertaining the analogy with thermodynamics, we provide a physical interpretation of model hyperparameters and propose analytical procedures for their estimate. These results enable the practical application of this novel and powerful framework to network model inference. We demonstrate this method in synthetic networks endowed with a modular structure, and in real-world brain connectivity networks.
Graph analysis and modularity of brain functional connectivity networks: searching for the optimal threshold
Bòrdier C., Nicolini C., Bifone A.
Frontiers in Neuroscience , (2017)
Abstract
Neuroimaging data can be represented as networks of nodes and edges that capture the topological organization of the brain connectivity. Graph theory provides a general and powerful framework to study these networks and their structure at various scales. By way of example, community detection methods have been widely applied to investigate the modular structure of many natural networks, including brain functional connectivity networks. Sparsification procedures are often applied to remove the weakest edges, which are the most affected by experimental noise, and to reduce the density of the graph, thus making it theoretically and computationally more tractable. However, weak links may also contain significant structural information, and procedures to identify the optimal tradeoff are the subject of active research. Here, we explore the use of percolation analysis, a method grounded in statistical physics, to identify the optimal sparsification threshold for community detection in brain connectivity networks. By using synthetic networks endowed with a ground-truth modular structure and realistic topological features typical of human brain functional connectivity networks, we show that percolation analysis can be applied to identify the optimal sparsification threshold that maximizes information on the networks’ community structure. We validate this approach using three different community detection methods widely applied to the analysis of brain connectivity networks: Newman’s modularity, InfoMap and Asymptotical Surprise. Importantly, we test the effects of noise and data variability, which are critical factors to determine the optimal threshold. This data-driven method should prove particularly useful in the analysis of the community structure of brain networks in populations characterized by different connectivity strengths, such as patients and controls.
Community detection in weighted brain connectivity networks beyond the resolution limit
Nicolini C., Bòrdier C., Bifone A.
NeuroImage 146, (2017)
Abstract
Graph theoretical methods provide a powerful framework to investigate brain connectivity networks and their modular organization. Recently, it has been shown that many graph-based methods suffer from a fundamental resolution limit that may have affected previous studies and prevented detection of modules that are smaller than an intrinsic scale. Optimization of Surprise, a resolution-limit-free function rooted in discrete probability theory, has revealed a substantially different picture. Indeed, a wide size distribution of modules, or communities, was found in binary networks, thus suggesting that a revision of the current models of the modular organization of brain connectivity may be in order. However, functional connectivity networks are intrinsically weighted, reflecting a continuous distribution of connectivity strengths between different brain regions. Here, we extend Surprise optimization to the study of weighted networks, and validate this new approach in synthetic networks endowed with a ground-truth modular structure. We compare Surprise with leading community detection methods currently in use and show its improved specificity and sensitivity in the detection of small modules even in the presence of noise and inter-subject variability such as those observed in fMRI experiments. Finally, we apply our novel approach to functional connectivity networks from resting state fMRI experiments, and demonstrate a heterogeneous modular organization, with a wide distribution of clusters spanning different scales.
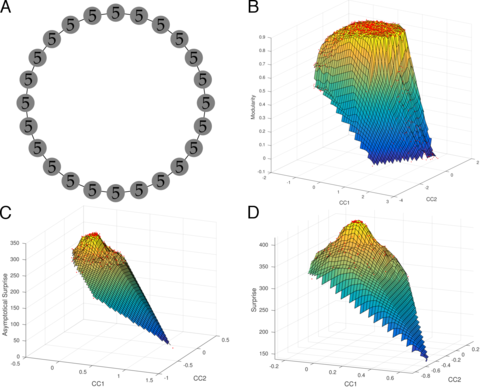
PubMed ID: 27865921
Modular structure of brain functional connectivity: breaking the resolution limit by Surprise
Nicolini C., Bifone A.
Scientific Reports 6, 19250, (2016)
Abstract
The modular organization of brain networks has been widely investigated using graph theoretical approaches. Recently, it has been demonstrated that graph partitioning methods based on the maximization of global fitness functions, like Newman’s Modularity, suffer from a resolution limit, as they fail to detect modules that are smaller than a scale determined by the size of the entire network. Here we explore the effects of this limitation on the study of brain connectivity networks. We demonstrate that the resolution limit prevents detection of important details of the brain modular structure, thus hampering the ability to appreciate differences between networks and to assess the topological roles of nodes. We show that Surprise, a recently proposed fitness function based on probability theory, does not suffer from these limitations. Surprise maximization in brain co-activation and functional connectivity resting state networks reveals the presence of a rich structure of heterogeneously distributed modules, and differences in networks’ partitions that are undetectable by resolution-limited methods. Moreover, Surprise leads to a more accurate identification of the network’s connector hubs, the elements that integrate the brain modules into a cohesive structure.
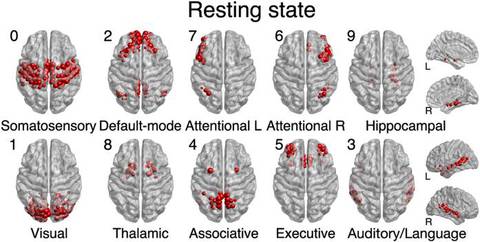
PubMed ID: 26763931
Disrupted modular organization of primary sensory brain areas in schizophrenics
Bordier C., Nicolini C., Forcellini G., Bifone A.
Neuroimage Clinical (2017)
Abstract
Abnormal brain resting-state functional connectivity has been consistently observed in patients affected by Schizophrenia (SCZ) using functional MRI and other neuroimaging methods. Graph theoretical methods provide a framework to investigate these defective functional interactions and their effects on the modular organization of brain connectivity networks. A few studies have shown reduced overall modularity in functional connectivity networks from SCZ patients, possibly an indication of an imbalance between functional segregation and integration. However, unambiguous identification of the neuroanatomical and functional substrates involved has proven elusive. We have recently demonstrated that current modularity analysis methods suffer from a fundamental resolution limit, as they fail to detect modules that are smaller than a scale determined by the size of the entire connectivity network. This resolution limit is likely to have affected previous studies and hampered the ability to resolve differences between patients and controls in cross-sectional studies. Here, we apply a novel, resolution- limit-free approach to study the modular organization of resting state functional connectivity networks in a cohort of SCZ patients, and in matched healthy controls. Leveraging these important methodological advances, we find evidence of substantial fragmentation and reorganization involving primary sensory, auditory and visual areas in SCZ patients. Conversely, frontal and prefrontal areas associated with higher cognitive functions appear to be less affected, with changes involving mostly language and speech processing areas. Our findings are consistent with the hypothesis that cognitive dysfunction in SCZ may arise from deficits occurring already at early stages of sensory processing.
A framework for the study of vision in active observers
Nicolini C., Fantoni A., Mancuso G., Volcic R., Domini F.
Proc. of SPIE Vol. 9014, 901414 , (2014)
Abstract
We present a framework for the study of active vision, i.e., the functioning of the visual system during actively self-generated body movements. In laboratory settings, human vision is usually studied with a static observer looking at static or, at best, dynamic stimuli. In the real world, however, humans constantly move within dynamic environments. The resulting visual inputs are thus an intertwined mixture of self- and externally-generated movements. To fill this gap, we developed a virtual environment integrated with a head-tracking system in which the influence of self- and externally-generated movements can be manipulated independently. As a proof of principle, we studied perceptual stationarity of the visual world during lateral translation or rotation of the head. The movement of the visual stimulus was thus parametrically tethered to self-generated movements. We found that estimates of object stationarity were less biased and more precise during head rotation than translation. In both cases the visual stimulus had to partially follow the head movement to be perceived as immobile. We discuss a range of possibilities for our setup among which the study of shape perception in active and passive conditions, where the same optic flow is replayed to stationary observers.
From on-going to complete activity recognition exploiting related activities
Nicolini C., Lepri B., Teso S., Passerini A.
Human Behavior Understanding, First International Workshop, HBU 2010, Istanbul, Turkey, August 22, 2010 (2010)
Abstract
Activity recognition can be seen as a local task aimed at identifying an on-going activity performed at a certain time, or a global one identifying time segments in which a certain activity is being per- formed. We combine these tasks by a hierarchical approach which locally predicts on-going activities by a Support Vector Machine and globally refines them by a Conditional Random Field focused on time segments in- volving related activities. By varying temporal scales in order to account for widely different activity durations, we achieve substantial improve- ments in on-going activity recognition on a realistic dataset from the PlaceLab sensing environment. When focusing on periods within which related activities are known to be performed, the refinement stage man- ages to exploit these relationships in order to correct inaccurate local predictions.
The modular structure of brain functional connectivity networks: a graph theoretical approach
Nicolini C.
PhD thesis University of Verona, (2017)
Abstract
Complex networks theory offers a framework for the analysis of brain functional connectivity as measured by magnetic resonance imaging. Within this approach the brain is represented as a graph comprising nodes connected by links, with nodes corresponding to brain regions and the links to measures of inter-regional interaction. A number of graph theoretical methods have been proposed to analyze the modular structure of these networks. The most widely used metric is Newman\ Modularity, which identifies modules within which links are more abundant than expected on the basis of a random network. However, Modularity is limited in its ability to detect relatively small communities, a problem known as resolution limit. As a consequence, unambiguously identifiable modules, like complete sub-graphs, may be unduly merged into larger communities when they are too small compared to the size of the network. This limit, first demonstrated for Newman Modularity, is quite general and affects, to a different extent, all methods that seek to identify the community structure of a network through the optimization of a global quality function. Hence, the resolution limit may represent a critical shortcoming for the study of brain networks, and is likely to have affected many of the studies reported in the literature. This work pioneers the use of Surprise and Asymptotical Surprise, two quality functions rooted in probability theory that aim at overcoming the resolution limit for both binary and weighted networks. Hereby, heuristics for their optimization are developed and tested, showing that the resulting optimal partitioning can highlight anatomically and functionally plausible modules from brain connectivity datasets, on binary and weighted networks. This novel approach is applied to the partitionining of two different human brain networks that have been extensively characterized in the literature, to address the resolution limit issue in the study of the brain modular structure. Surprise maximization in human resting state networks revealed the presence of a rich structure of modules with heterogeneous size distribution undetectable by current methods. Moreover, Surprise led to different, more accurate classification of the network connector hubs, the elements that integrate the brain modules into a cohesive structure. In synthetic networks, Asymptotical Surprise showed high sensitivity and specificity in the detection of ground-truth structures, particularly in the presence of noise and variability such as those observed in experimental functional MRI data. Finally, the methodological advances hereby introduced are shown to be an helpful tool to better discern differences between the modular organization of functional connectivity of healthy subjects and schizophrenic patients. Importantly, these differences may point to new clinical hypotheses on the aetiology of schizophrenia, and they would have gone unnoticed with resolution-limited methods. This may call for a revisitation of some of the current models of the modular organization of the healthy and diseased brain.
Conferences and proceedings
– Carlo Nicolini, Thermodynamic of network model fitting with spectral entropies. NETSCI2018, Paris, June 11-16th 2018. pdf
-
Carlo Nicolini, Cècile Bordier, Angelo Bifone, “Community detection in weighted brain connectivity networks beyond the resolution limit”, Conference of the Complex Systems Society, Amsterdam, (2016) CCS2016.
-
Carlo Nicolini, Adam Liska, Francesco Sforazzini, Alberto Galbusera, Angelo Bifone, Alessandro Gozzi. Modular organization of mouse brain functional connectivity networks. ISMRM Joint Annual Meeting, Milano (2014).
-
Carlo Nicolini, Carlo Fantoni, Giovanni Mancuso, Robert Volcic, Fulvio Domini. A framework for the study of vision in active observers. IS&T SPIE Electronic Imaging, San Francisco (2014).
-
Walter Gerbino, Carlo Fantoni, Carlo Nicolini, Robert Volcic, Fulvio Domini. Active Multisensory Perception Tool: BUS experience and action comfort. Human Factors and Ergonomics Society Europe, Torino (2013).
-
Carlo Nicolini, Stefano Teso, Bruno Lepri, Andrea Passerini. From on-going to complete activity recognition exploiting related activities. International Workshop on Human Behaviour Understanding, Istanbul (2010).
Summer schools
- Complex networks and their applications, May 15, 2016, Como Villa del Grumello.
- NSAS Connectomics summer school, May 20 2015 Villa Finaly, Florence